Note
Go to the end to download the full example code.
Alpha CSC on simulated data#
This example demonstrates alphaCSC [1] on simulated data.
# Authors: Mainak Jas <mainak.jas@telecom-paristech.fr>
# Tom Dupre La Tour <tom.duprelatour@telecom-paristech.fr>
# Umut Simsekli <umut.simsekli@telecom-paristech.fr>
# Alexandre Gramfort <alexandre.gramfort@telecom-paristech.fr>
#
# License: BSD (3-clause)
Let us first define the parameters of our model.
Next, we define the parameters for alpha CSC
n_iter_global = 10
n_iter_optim = 50
n_iter_mcmc = 100
n_burnin_mcmc = 50
Here, we simulate the data
from alphacsc.simulate import simulate_data
random_state_simulate = 1
X, ds_true, z_true = simulate_data(n_trials, n_times, n_times_atom,
n_atoms, random_state_simulate)
Add some noise and corrupt some trials even with impulsive noise
from scipy.stats import levy_stable
from alphacsc import check_random_state
fraction_corrupted = 0.02
n_corrupted_trials = int(fraction_corrupted * n_trials)
# Add stationary noise:
rng = check_random_state(random_state_simulate)
X += 0.01 * rng.randn(*X.shape)
noise_level = 0.005
# add impulsive noise
idx_corrupted = rng.randint(0, n_trials,
size=n_corrupted_trials)
X[idx_corrupted] += levy_stable.rvs(alpha, 0, loc=0, scale=noise_level,
size=(n_corrupted_trials, n_times),
random_state=random_state_simulate)
and then alpha CSC on the same data
from alphacsc import learn_d_z_weighted
d_hat, z_hat, Tau = learn_d_z_weighted(
X, n_atoms, n_times_atom, reg=reg, alpha=alpha,
solver_d_kwargs=dict(factr=100), n_iter_global=n_iter_global,
n_iter_optim=n_iter_optim, init_tau=True,
n_iter_mcmc=n_iter_mcmc, n_burnin_mcmc=n_burnin_mcmc,
random_state=60, n_jobs=1, verbose=1)
Global Iter: 0/10 V_0/50 /github/workspace/alphacsc/update_d.py:172: DeprecationWarning: Conversion of an array with ndim > 0 to a scalar is deprecated, and will error in future. Ensure you extract a single element from your array before performing this operation. (Deprecated NumPy 1.25.)
lambd_hats[k] = lambd_hat
.................................................
Global Iter: 1/10 V_0/50 .................................................
Global Iter: 2/10 V_0/50 .................................................
Global Iter: 3/10 V_0/50 .................................................
Global Iter: 4/10 V_0/50 .................................................
Global Iter: 5/10 V_0/50 .................................................
Global Iter: 6/10 V_0/50 .................................................
Global Iter: 7/10 V_0/50 .................................................
Global Iter: 8/10 V_0/50 .................................................
Global Iter: 9/10 V_0/50 .................................................
Finally, let’s compare the results. Now, it works even in the presence of impulsive noise.
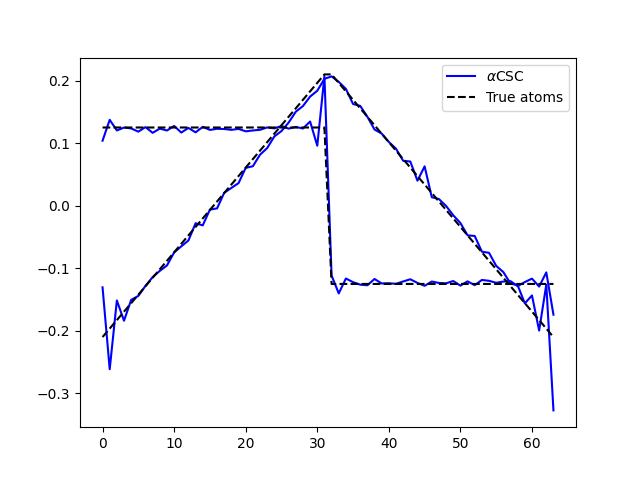
We can even visualize the weights to see what time points were downweighted by the algorithm
fig, axes = plt.subplots(2, 1, sharex=True)
axes[0].set_xlim([-20, n_times])
axes[0].set_ylim([0, 2])
axes[1].set_ylim([-0.5, 0.3])
for t in [2, 250]:
axes[0].axvline(t, linestyle='-.')
axes[1].axvline(t, linestyle='-.')
axes[0].plot(1 / Tau[idx_corrupted[0], :])
axes[0].set_ylabel('1 / weights')
axes[1].plot(X[idx_corrupted[0], :])
axes[1].set_ylabel('Corrupted trial')
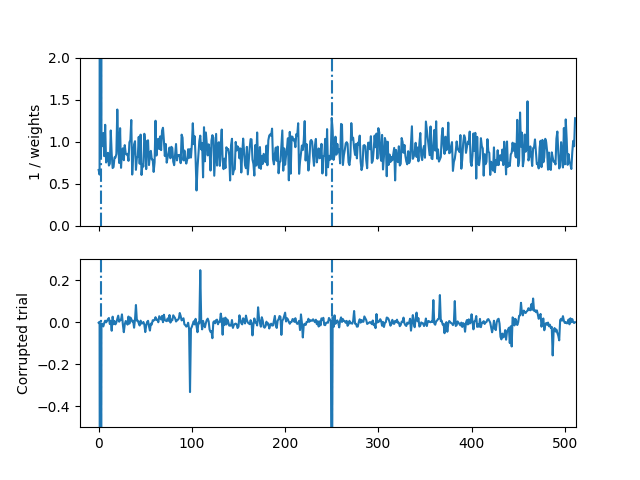
Text(31.222222222222214, 0.5, 'Corrupted trial')
Total running time of the script: (3 minutes 27.496 seconds)